RBLNServe (모델서버)
RBLN SDK는 REST 및 gRPC 프로토콜 기반의 모델 서빙 프레임워크인 RBLNServe를 제공합니다. RBLN 런타임을 직접 다룰 필요없이 RBLNServe를 사용하여 웹기반의 인터페이스를 통해 쉽게 모델 추론을 수행할 수 있고, 다른 웹서비스들과도 쉽게 통합할 수 있습니다.
설치
시작하기에 앞서 시스템에 아래 항목들이 준비되어 있는지 확인합니다:
- 우분투 20.04 LTS (Debian bullseye) 이상
- RBLN NPU가 장착되어있는 서버(예, 리벨리온 ATOM NPU)
- 파이썬(3.9 - 3.12 지원)
- RBLN SDK (드라이버, 컴파일러)
위 항목이 준비되어있는 시스템에 RBLNServe를 설치합니다. RBLNServe 설치를 위해 리벨리온 사설 PyPI 서버 접근 권한이 필요합니다:
| $ pip install -i https://pypi.rbln.ai/simple rblnserve
|
사용법
커맨드 라인 인터페이스
RBLNServe 설치가 완료되었다면 rblnserve
커맨드 라인 인터페이스(Command Line Interface) 유틸리티를 사용할 수 있습니다:
| $ rblnserve --help
Usage: rblnserve [OPTIONS]
Options:
--host TEXT IPv4 address to bind
--rest-port INTEGER REST server port to listen
--grpc-port INTEGER GRPC server port to listen
--config-file TEXT path to model config yaml file
--version print RBLNServe version
--install-completion [bash|zsh|fish|powershell|pwsh]
Install completion for the specified shell.
--show-completion [bash|zsh|fish|powershell|pwsh]
Show completion for the specified shell, to
copy it or customize the installation.
--help Show this message and exit.
|
모델 서빙
아래의 예시를 통해 RBLNServe 구동을 위한 컴파일된 모델을 준비합니다(컴파일 방법):
| import torch
import torchvision
import rebel
# 컴파일된 모델 준비하기 - 이 예시에서는 'resnet50.rbln'
model_name = "resnet50"
input_name = "input0"
input_shape = [1,3,224,224]
weights = torchvision.models.get_model_weights(model_name).DEFAULT
model = getattr(torchvision.models, model_name)(weights=weights).eval()
compiled_model = rebel.compile_from_torch(model, [(input_name, input_shape, torch.float32)])
compiled_model.save(model_name+".rbln")
|
컴파일된 모델 /path/to/your/model/resnet50.rbln
을 기반으로 아래와 같은 설정파일(model_config.yaml
)을 생성할 수 있습니다. 여러 개의 모델 구성을 추가하여 다수의 모델을 동시에 서빙할 수도 있습니다.
| models:
- name: my-model
path: "/path/to/your/model/resnet50.rbln"
version: 0.1.0 #optional
description: "ResNet50 Test" #optional
|
설정파일이 준비되면 아래 명령어를 통해 서버를 구동할 수 있습니다:
| $ rblnserve --config-file=model_config.yaml
____________ _ _ _ _____
| ___ \ ___ \ | | \ | |/ ___|
| |_/ / |_/ / | | \| |\ `--. ___ _ ____ _____
| /| ___ \ | | . ` | `--. \/ _ \ '__\ \ / / _ \
| |\ \| |_/ / |____| |\ |/\__/ / __/ | \ V / __/
\_| \_\____/\_____/\_| \_/\____/ \___|_| \_/ \___|
2023-08-16 09:46:58,809 INFO: model has been loaded: name='my-model' path='/path/to/your/model/resnet50.rbln' version='0.1.0' description='ResNet50 Test'
2023-08-16 09:46:58,822 INFO: Started server process [38167]
2023-08-16 09:46:58,822 INFO: Waiting for application startup.
2023-08-16 09:46:58,825 INFO: GRPC server listening on 0.0.0.0:8081
2023-08-16 09:46:58,825 INFO: Application startup complete.
2023-08-16 09:46:58,825 INFO: Uvicorn running on http://0.0.0.0:8080 (Press CTRL+C to quit)
|
REST API 기반 추론 요청
추론 요청을 위해 페이로드 스펙을 참고하여 아래와 같이 json 페이로드를 준비합니다:
| import urllib.request
import json
import torchvision
model_name = "resnet50"
input_name = "input0"
input_shape = [1,3,224,224]
weights = torchvision.models.get_model_weights(model_name).DEFAULT
preprocess = weights.transforms()
# json 페이로드 준비하기 - 이 예시에서는 'tabby.json'
img_url = "https://rbln-public.s3.ap-northeast-2.amazonaws.com/images/tabby.jpg"
response = urllib.request.urlopen(img_url)
with open("tabby.jpg", "wb") as f:
f.write(response.read())
img = torchvision.io.image.read_image("tabby.jpg")
img = preprocess(img).unsqueeze(0).numpy()
input_data = img.flatten().tolist()
payload = {
"inputs": [
{
"name": input_name,
"shape": input_shape,
"datatype": "FP32",
"data": input_data
}
]
}
with open("tabby.json", "w") as f:
json.dump(payload, f)
|
아래의 방법들 중 하나를 사용하여 준비된 json 페이로드(/path/to/your/payload/tabby.json
)에 대한 추론 요청을 보낼 수 있습니다:
Python requests
| import requests
import json
url = "http://localhost:8080/v2/models/my-model/versions/0.1.0/infer"
with open("/path/to/your/payload/tabby.json", "r") as f:
payload = json.load(f)
response = requests.post(url, json=payload)
print(response.json())
|
NodeJS axios
| var axios = require("axios");
var fs = require("fs");
var payload = fs.readFileSync("/path/to/your/payload/tabby.json");
var config = {
method: "post",
url: "http://localhost:8080/v2/models/my-model/versions/0.1.0/infer",
headers: {
"Content-Type": "application/json"
},
data: payload
}
axios(config)
.then(function (response) {
console.log(response.data);
})
.catch(function (error) {
console.error(error);
})
|
curl
| $ curl -X POST -H "Content-Type: application/json" -d "@/path/to/your/payload/tabby.json" http://localhost:8080/v2/models/my-model/versions/0.1.0/infer
|
gRPC API 기반 추론 요청
gRPC API 사용을 위해 KServe grpc 프로토 파일 기반의 pb2 코드를 생성해야 합니다. gRPC 가이드 문서를 참고하여 파이썬 혹은 사용하는 언어에 맞는 pb2 코드를 생성하시기 바랍니다. 직접 pb2 코드를 생성하지 않고 rblnserve
에서 제공하는 pb2 모듈인 rblnserve.api.grpc.predict_v2_pb2
와 rblnserve.api.grpc.predict_v2_pb2_grpc
를 사용할 수도 있습니다.
위와 같이 입력 데이터가 준비되었다면, 아래의 방법들 중 하나(혹은 gRPC를 지원하는 또다른 언어 및 라이브러리)를 사용하여 추론 요청을 보낼 수 있습니다:
Python grpcio
| import grpc
# import your generated pb2 files
import your.modules.generated_pb2 as pb2
from your.modules.generated_pb2_grpc import GRPCInferenceServiceStub
channel = grpc.insecure_channel("localhost:8081") # replace with your rblnserve grpc endpoint
stub = GRPCInferenceServiceStub(channel)
# prepare your preprocessed inputs
input_data = ...
request = pb2.ModelInferRequest(
model_name="my-model",
model_version="0.1.0",
inputs=[
pb2.ModelInferRequest.InferInputTensor(
name="input0",
shape=[1, 3, 224, 224],
datatype="FP32",
contents=pb2.InferTensorContents(fp32_contents=input_data),
)
],
)
response = stub.ModelInfer(request)
|
NodeJS grpc-js
| const grpc = require("@grpc/grpc-js");
const protoLoader = require("@grpc/proto-loader");
const packageDefinition = protoLoader.loadSync("path_to_proto_file.proto", {
keepCase: true,
longs: String,
enums: String,
defaults: true,
oneofs: true,
});
const protoDescriptor = grpc.loadPackageDefinition(packageDefinition);
const service =
protoDescriptor.your.modules.generated_pb2_grpc.GRPCInferenceService;
const client = new service(
"localhost:8081", // replace with your rblnserve grpc endpoint
grpc.credentials.createInsecure()
);
// prepare your preprocessed inputs
const input_data = ...
const request = {
modelName: "my-model",
modelVersion: "0.1.0",
inputs: [
{
name: "input0",
shape: [1, 3, 224, 224],
datatype: "FP32",
contents: { fp32Contents: input_data },
},
],
};
client.ModelInfer(request, (error, response) => {
if (error) {
console.error(error);
return;
}
console.log(response);
});
|
API 엔드포인트
RBLNServe가 제공하는 API 엔드포인트는 KServe Predict Protocol V2를 준수 합니다.
REST API
endpoint |
description |
GET /v2 |
Returns server metadata (link) |
GET /v2/health/live |
Returns server liveness (link) |
GET /v2/health/ready |
Returns server readiness (link) |
GET /v2/models/{MODEL_NAME} |
Returns a model metadata specified by MODEL_NAME (link) |
GET /v2/models/{MODEL_NAME}/ready |
Returns a model readiness specified by MODEL_NAME (link) |
POST /v2/models/{MODEL_NAME}/infer |
Returns inference results of a model specified by MODEL_NAME (link) |
GET /v2/models/{MODEL_NAME}/versions/{MODEL_VERSION} |
Returns a model metadata specified by MODEL_NAME and MODEL_VERSION (link) |
GET /v2/models/{MODEL_NAME}/versions/{MODEL_VERSION}/ready |
Returns a model readiness specified by MODEL_NAME and MODEL_VERSION (link) |
POST /v2/models/{MODEL_NAME}/versions/{MODEL_VERSION}/infer |
Returns inference results of a model specified by MODEL_NAME and MODEL_VERSION (link) |
http://localhost:8080/docs
를 통해 접근할 수 있는 Swagger 문서를 참고하여 REST API를 검토하고 테스트할 수 있습니다:
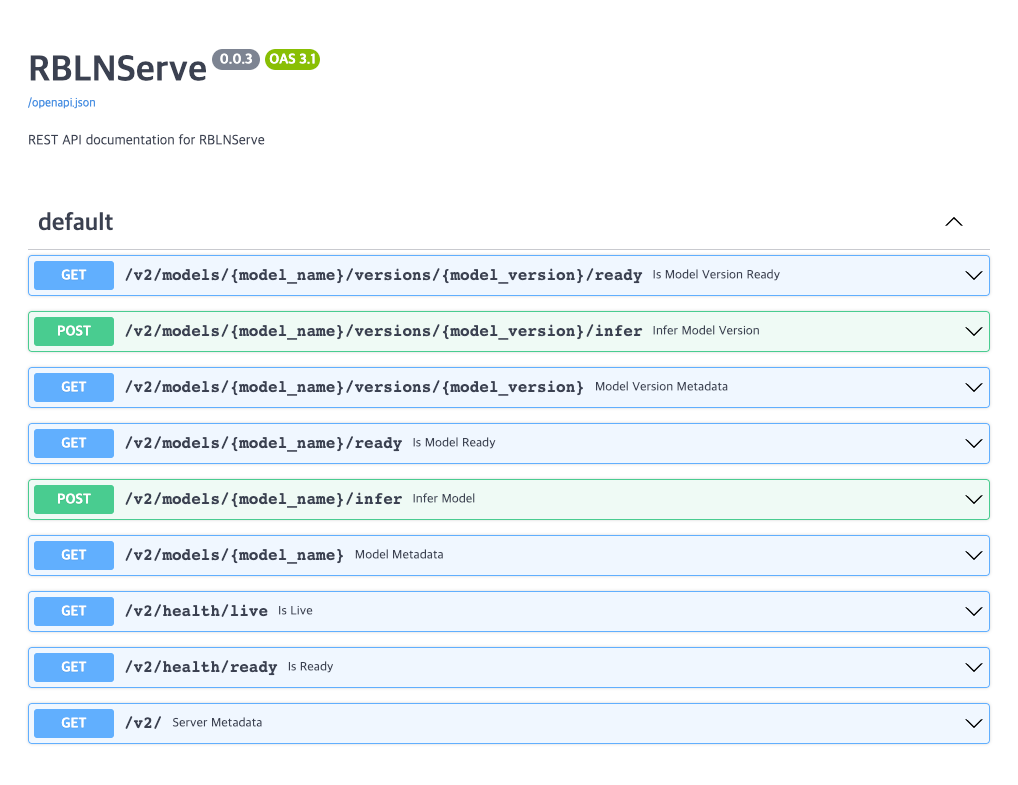
gRPC API
rpc |
description |
ServerMetadata |
Returns server metadata (link) |
ServerLive |
Returns server liveness (link) |
ServerReady |
Returns server readiness (link) |
ModelMetadata |
Returns a model metadata specified by name and version (link) |
ModelReady |
Returns a model readiness specified by name and version (link) |
ModelInfer |
Returns inference results of a model specified by name and version (link) |
RBLNServe gRPC 서버는 server reflection을 지원하기 때문에 Postman과 같은 도구를 사용하여 서비스 정의(service definition)를 검토할 수 있습니다.